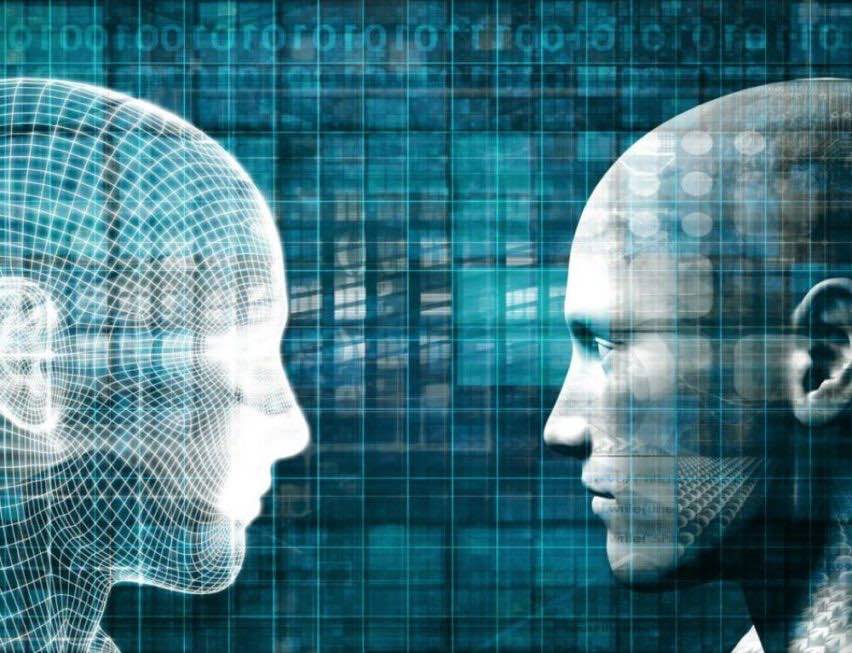
AI Predicts Death? Stanford researchers have developed an AI that can predict when a patient will die with up to 90 percent accuracy.
Using artificial intelligence to predict when patients may die sounds like an episode from the dystopian science fiction TV series “Black Mirror.” But Stanford University researchers see this use of AI as a benign opportunity to help prompt physicians and patients to have necessary end-of-life conversations earlier.
Many physicians often provide overly rosy estimates about when their patients will die and delay having the difficult conversations about end-of-life options. That understandable human tendency can lead to patients receiving unwanted, expensive and aggressive treatments in a hospital at their time of death instead of being allowed to die more peacefully in relative comfort. The alternative being tested by a Stanford University team would use AI to help physicians screen for newly-admitted patients who could benefit from talking about palliative care choices.
Past studies have shown that about 80 percent of Americans would prefer to spend their last days at home if possible. In reality, up to 60 percent of Americans end up dying in an acute care hospital while receiving aggressive medical treatments, according to research cited by the Stanford group’s paper “Improving Palliative Care with Deep Learning” published on the arXiv preprint server.
Palliative care experts usually wait for the medical team in charge of a given patient to request their services, which typically include providing relief for patients suffering from serious illnesses and possibly recording end-of-life treatment preferences in a living will. But Stephanie Harman, an internal medicine physician and founding medical director of Palliative Care Services for Stanford Health Care, saw an opportunity to flip that routine around by giving palliative care physicians the ability to identify and proactively reach out to patients.
Harman took her idea to Nigam Shah, associate professor of medicine and biomedical informatics at Stanford University. Shah had been talking about possible collaborations involving AI in healthcare with Andrew Ng, an adjunct professor at Stanford University and former head of the Baidu AI Group. They agreed that the palliative care idea seemed like a good project to explore together.
The Stanford team’s AI algorithms rely upon deep learning, the popular machine learning technique that uses neural networks to filter and learn from huge amounts of data. The researchers trained a deep learning algorithm on the Electronic Health Records of about 2 million adult and child patients admitted to either the Stanford Hospital or Lucile Packard Children’s hospital to predict the mortality of a given patient within the next three to 12 months. (Predicting the death of a patient within three months would provide too little time for the preparations needed in palliative care.)
”We could build a predictive model using routinely collected operational data in the healthcare setting, as opposed to a carefully designed experimental study,” says Anand Avati, a PhD candidate in computer science at the AI Lab of Stanford University. “The scale of data available allowed us to build an all-cause mortality prediction model, instead of being disease or demographic specific.”
The pilot study’s use of an algorithm to predict patient mortality—which was approved by an institutional review board—turns out to be less scary than one might think. From an ethics and medical care standpoint, the deep learning model’s assistance in helping human physicians screen patients for palliative care generally comes with major benefits and few downsides.
“We think that keeping a doctor in the loop and thinking of this as ‘machine learning plus the doctor’ is the way to go as opposed to blindly doing medical interventions based on algorithms… that puts us on firmer ground both ethically and safety-wise,” says Kenneth Jung, a research scientist at Stanford University.
One potential complication with deep learning algorithms is that even their creators often cannot explain why a deep learning model came up with a particular result. That black box nature of deep learning means it might normally be difficult to tell how the Stanford group’s model comes to the conclusion that any given patient would likely die within a year.
Fortunately, the reasoning behind the deep learning model’s mortality predictions does not particularly matter in this case. The palliative care team is primarily concerned with accurately identifying patients who could benefit from their attention, as opposed to needing to know exactly why the algorithm predicts a given patient might die within a year. Jung explains it as follows:
That’s why in this particular case we’re more comfortable with having a black box model. The palliative care intervention is not tied to why somebody is getting sick. If it was a different hypothetical case of ‘somebody is going to die and we need to pick treatment options,’ in that case we do want to understand the causes because of the treatment. But in this setting, it doesn’t matter as much as long as we get it right.
Still, it may be useful to know why the deep learning model made its predictions for research purposes. In this case, the Stanford group used a common error analysis technique, called ablation analysis, to provide some insight behind the deep learning model’s decision-making. Their method involved tuning the model little by little through tweaking individual parameters to figure out what impact those parameters had on the model’s decisions.
The Stanford group also emphasized that patients do not need to be standing near death’s door in order to benefit from palliative care. The early stages of the pilot study showed that it was often beneficial for physicians to have the end-of-life discussions with seriously ill patients even if they were not likely to die within the next year, Jung says.
In the end, the deep learning model’s focus on predicting death is far from sinister. Mortality simply happens to be a useful measure that is fairly straightforward—is the person dead or not—compared with the researchers’ main interest in figuring out the best timing for patients to get a visit from the palliative care team.
The Stanford group aims to gauge the pilot study’s success based on outcomes such as how the physicians on both the palliative care team and the first-line team caring for the patients behave differently. They also want to see if the AI prescreening can improve the rate of patients getting their wishes for end-of-life care documented and reduce the number of people who end up dying in the intensive care unit (ICU) against their interests.
“We want to make sure the sickest patients and their families get a chance to talk about what they want to happen before they become critically ill and they end up in the ICU,” Jung says.